The Brown Center invites you to our Faculty Spotlight on Wednesday, February 3rd at 12:00 PM on Blackboard Ultra. The spotlight series is a showcase of research, creative inquiry, and other scholarly engagement of the campus community. Stop by and learn about the research of our talented Stetson community!
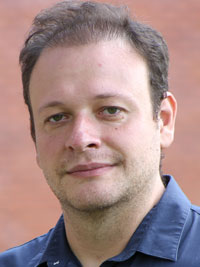
Dr. Petros Xanthopoulos – Associate Professor of Decision & Information Sciences and Exec. Dir. of Graduate Programs
Department of Decision and Information Sciences
A minimum variance ensemble clustering algorithm based on modern portfolio theory
In analytics, the problem of clustering refers to the classification of similar objects in different groups with the ultimate goal to understand the specific characteristics of each group. Since the discovery of one of the most commonly used clustering algorithms in 1967, there has been a rich literature in diverse methods and algorithms, each targeting problems and datasets with different characteristics and class distributions. Even today there is no de facto best algorithm for every dataset simply because each method is usually designed to perform better in particular class of problems. For this, there has been a significant amount of literature proposing method- ologies for combing different clustering algorithms with the expectation to come up with a clustering with superior performance. This methodology is called consensus clustering or unsupervised ensemble learning. In this presentation we propose a method for consensus clustering methodology by taking into consideration the intra algorithmic variability i.e. algorithms that provide solutions with very different performance upon multiple runs. The methodology is inspired by modern portfolio theory and more specifically from Markowitz minimum variance asset allocation.
Petros Xanthopoulos, PhD, earned his Diploma of Engineering from the Technical University of Crete in Electronics and Computer Engineering. He earned his MSc and PhD in Industrial and Systems Engineering from University of Florida. Dr. Xanthopoulos’ research focuses on analytics, machine learning, and operations research with an emphasis on development of data analysis methods for data under uncertainty. He has authored one monogram, edited three books, and authored more than thirty peer reviewed journal articles. He has taught courses in Statistics, Managerial Decision Analytics, and Machine Learning for Business Decisions in both undergrad and MBA programs. He serves as associate editor for the journals Expert Systems with Applications (Elsevier), Optimization Letters (Springer) and Energy Systems (Springer). He has been a member of the Institute for Operations Research and Management Science (INFORMS) since 2007.